Investor’s Intention to Adopt Online Stock Trading in Nepal
It is found that performance expectancy and effort expectancy are positively and significantly related to behavior intention.
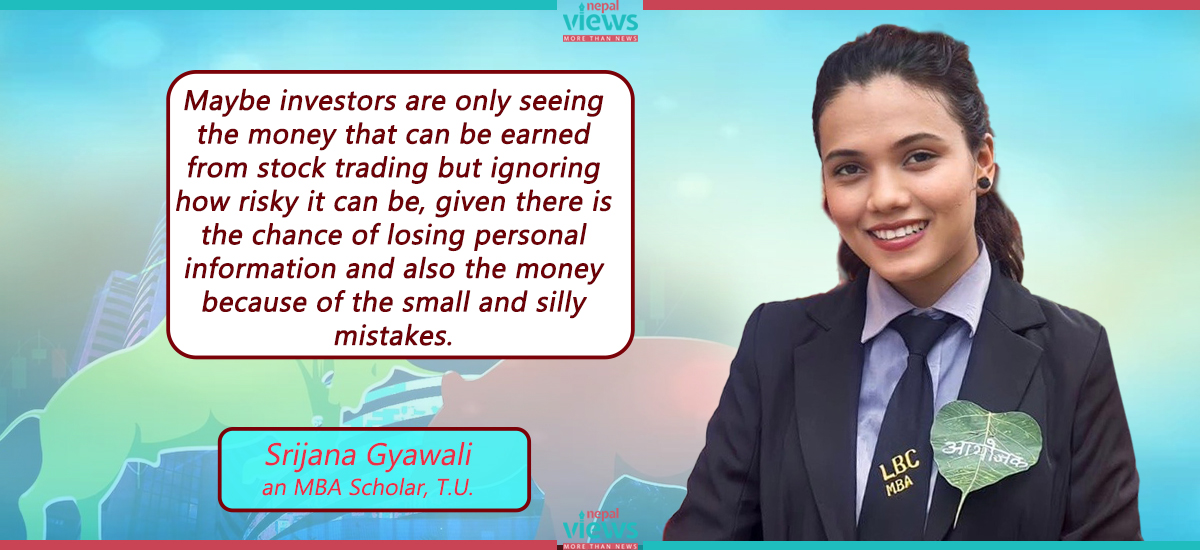
ABSTRACT
This study examines the effect of performance expectancy, effort expectancy, social influence, security risk, economic risk, functional risk, and investor’s intention to adopt online stock trading. This research is based on the primary data. The primary source of the data was used to study the factors that influence the investor’s intention to adopt online stock trading in Nepal. To collect the responses to the study and get into the research objectives, 89 respondents were selected as the sample size. Data were collected with the help of a structured questionnaire which was further subjected to the Reliability test, Normality test, and correlation regression analysis of respondents in SPSS. Results show that Performance Expectancy and Effort Expectancy have a high degree of positive correlation with Behavioral Intention, while Social Influence, Security Risk, Economic Risk, and Functional Risk have a moderate degree of positive correlation with Behavioral Intention.
Key Words: NEPSE, Trading system, online trading, investors
INTRODUCTION
There must be change when something is unchangeable. The Greek philosopher Heraclitus once remarked that “Change is the only constant in life.” The world, which is thought to have originated some 4.54 billion years ago, has continued to change and is now in the twenty-first century. The age of technology from the Stone Age has arrived in the modern world. The advancement of technology has fundamentally altered the planet. Technology has given this planet new forms, from a basic wristwatch to satellites, from a calculator to airplanes. Artificial Intelligence (AI) is one of technology’s greatest contributions to the world. We no longer need to go shopping because everything can be ordered online and delivered right to our house. We can do all of those banking transactions while sitting at home instead of having to go to the bank. Every action we perform now is easy, practical, and affordable thanks to technology. The technological revolution has affected every industry in the world, including food, retail, sports, and education.
Now is easy, practical, and affordable thanks to technology. The technological revolution has affected every industry in the world, including food, retail, sports, and education.
The 21st century has so far been the age of technology and science. Today’s population uses computerized personal transportation, drives intelligent automobiles, does business on a smartphone, and has a persistent internet connection. Through the ages, technology has helped humans accomplish more in less time and with less effort. This is hailed by many as a means to simplify living. The way of life for people has never been the same since the technology revolution began. Technology has not only made life simpler for individuals, but it has also opened up new economic prospects. The power of technology to help businesses accomplish more with less and provide better results is accelerating, in particular thanks to the relationships between artificial intelligence (AI), big data, and the Internet of Things (IoT) (Wilburn, 2018).
The major objective of the study is to investigate the Investor’s intention to adopt online stock trading the specific objectives of the study are stated as follows.
- To measure the relationship between performance expectancy, effort expectancy, social influence, security risk, economic risk, functional risk, and investor’s intention to adopt online stock trading.
- To examine the effect of performance expectancy, effort expectancy, social influence, security risk, economic risk, functional risk, and investors’ intention to adopt online stock trading
LITERATURE REVIEW
Bagozzi and Dholakia (2002) carried out a study to investigate the individual and social determinants of the members’ intentions to participate in virtual communities. An empirical study on 157 regular virtual community participants using multiple regression analysis finds that intentions to participate in virtual communities are functions of both individual determinants (positive anticipated emotions and desires) and community influences (social identity).
Constantiou & Mahnke, (2010) also carried out a study to explore the relationship between the individual’s perceptions of value elements and the adoption intentions, in the case of mobile TV. They interviewed 30 young adults working in the private sector, or students in Austria and also collected questionnaires from 232 respondents, through the analysis, they concluded that males tend to disregard others’ opinions, while females tend to view the opinions of others as opportunities to enhance their decision-making capabilities.
Talukder, (2012) also investigated the determinants of the adoption of technological innovation by individual employees within an organizational context in Australia using TRA and TAM as the basis of the theoretical framework. The data from 275 respondents from an Australian organization through a survey questionnaire. When they analyzed the collected data through correlation and regression analysis, the results showed that individual adoption of innovation is influenced by two social factors – peers and social networks.
Performance expectancy is largely determined by indicators such as perceived usefulness, intrinsic and extrinsic motivation, job fit, relative advantage, and outcome expectations of Information Technology (Wu, Yu, & Weng, 2012).
Maharjan (2018) carried out a study to explore citizens’ behavior intention toward the adoption of e-government services in Nepal using the Unified Theory of Acceptance and Use of Technology model. This study performed a field survey of 213 people and used Structural Equation Modeling to evaluate the findings. The result revealed that the effect of success expectation, effort expectation, social influence, confidence, and website efficiency on usage behavior was not moderated by Behavior Intention.
Poudel (2018) also studied the behavioral intention of job seekers to use online recruitment services in the Nepalese context based on five determinants: Performance expectancy, Effort expectancy, Subjective Norms, Objective Norms, and Facilitating conditions. A total of 56 respondents were selected using a purposeful sampling process consisting of students at the final year master level studying at two Kathmandu business schools and evaluated using multiple regression analysis, which found that only success expectation and commitment expectation have a positive effect on the behavioral intention of job seekers to use online recruitment services.
In contrast, the findings of a study (Thaker et al., 2019) to analyze the factors that affect the behavioral intention of investors to invest in a P2P lending network, where the study was focused on an expanded technology adoption model and a formal survey questionnaire, showed that intention to invest is primarily influenced or motivated by confidence, with protection does not affect intention investor’s decisions.
THEORETICAL FRAMEWORK
The Theoretical framework is developed from the literature review discussed. This diagram is the foundation on which the entire research work is based. It explains the relationship between dependent variables and explanatory variables.
Theoretical Framework of the Study In Figure 1, the dependent variable is behavioral intention to adopt online stock trading while the independent variables are positive determinants (performance expectancy, effort expectancy, and social influence), and negative determinants (security risk, economic risk, and functional risk).
RESEARCH METHODOLOGY
This study is descriptive. It aims to draw a picture describing the investor’s intention to adopt online stock trading. I use the Cross-Sectional research method as the data was collected at a single point in time. Further, a descriptive research design is suitable as primary data is collected from a sample of respondents, and the findings of the study are generalized to represent the whole population where the sample was drawn from. Cross-Sectional research design is used to examine the effect of performance expectancy, effort expectancy, social influence, security risk, economic risk, functional risk, and investor’s intention to adopt online stock trading. To collect the responses to the study and get into the research objectives, 89 respondents were selected as the sample size.
Data Collection Procedures
This research is based on the primary data. The primary source of the data was used to study the factors that influence the investor’s intention to adopt online stock trading in Nepal.
For the study, primary data was collected, processed, and analyzed. However, the secondary information helped in the construction of the theoretical framework, questionnaire, and sampling design. Primary data was collected through a self-administered questionnaire. A questionnaire survey was conducted on individual investors. Each of the independent variables i.e. performance expectancy, effort expectancy, social influence, security risk, economic risk, and operational risk had 4 constructs each, and the dependent variable i.e. behavioral intention to adopt online stock trading had 3 constructs. All the constructs were measured on a 5-point Likert scale. The questionnaire was distributed electronically using a Google form.
Data analysis
Descriptive Statistics was used to summarize the data collected from the questionnaire. The study also uses Inferential Statistics, including correlation, and regression analysis, to examine the relationship between Performance Expectancy and Behavioral Intention. The statistical software package Statistical Package for Social Sciences (SPSS) was used to analyze the data.
The information from Table 2 indicates that 64 percent of participants were male, while 36 percent were female. The majority of respondents 57.2 percent were in the 18-25 age category, followed by 29 percent in the 26-35 age category, and 13.8 percent in the 36 or older category. In terms of occupation, 45.5 percent of the respondents were students, 35.2 percent were salaried employees, and the remaining 19.3 percent were either professionals or businesspeople. Regarding online stock trading, 93.1 percent of the sampled respondents currently use it, indicating its popularity among investors, while 6.9 percent do not use it for various reasons.
Table 3: Analysis of Determinants of Online Stock Trading
Mean | Std. Deviation | |
Better performance |
1.57 | .944 |
Ease of use |
1.98 | 1.011 |
Social influence |
2.50 | 1.174 |
System security |
2.59 | 1.172 |
Low cost of maintenance |
2.73 | 1.122 |
Service reliability |
2.76 |
1.295 |
Table 3 shows The First position is Better Performance with the least mean of 1.57 and standard deviation of 0.944, followed by better performance, system security, service reliability, peer influence, and maintenance cost respectively with means of 1.98,2.50, 2.59,2.73 and 4.49 and standard deviation of 1.011, 1.174, 1.172, 1.122 and 1.295.
Opinions on Factors Influencing Adaptation of Online Stock Trading
Table 4: Analysis of Performance Expectancy
Statements | Mean | Std. Deviation | |
Using online stock trading would enhance my stock trading efficiency. | 3.90 | 1.139 | |
I feel online stock trading is useful. | 3.99 | 1.046 | |
Using online stock trading would enable me to increase the convenience of stock trading. | 3.87 | 1.076 | |
Using online stock trading would enable me to accomplish stock trading more quickly. | 3.79 | 1.069 | |
Performance Expectancy | 3.88 | 1.08 |
Table 4 shows the strongest factor is perceived usefulness with an average mean of 3.99 and the least strong is perceived efficiency enhancement with an average mean of 3.79. The average is 3.88which is higher than the mean value of 3. This shows that most people expect online stock trading to enhance their performance related to stock trading.
Table 5: Analysis of Effort Expectancy
Statements | Mean | Std. Deviation |
Learning how to use mobile stock trading would be easy for me. | 3.61 | 1.108 |
I expect to find online stock trading clear and stable. | 3.53 | 1.039 |
t would be easy for me to become skillful at using online stock trading. | 3.61 | 1.060 |
I would find online stock trading easy to use. | 3.64 | 1.052 |
Effort Expectancy | 3.59 | 1.06 |
Table 5 shows the strongest factor is perceived usefulness with an average mean of 3.64 and the least strong is perceived easiness of developing online trading skills with an average mean of 3.53. The average is 3.659 which is higher than the mean value of 3. This shows that most people expect online stock trading to save their trading efforts while trading through online stock trading.
Table 6: Analysis of Social Influence
Statements | Mean | Std. Deviation |
I feel people around me would encourage me to use online stock trading. | 3.61 | 1.217 |
I will discuss online stock trading with my peers. | 3.55 | .925 |
In my environment, people who use online stock trading have more prestige than those who do not. | 2.97 | 1.011 |
e who are important to me would think that I should use online stock trading. | 3.32 | 1.006 |
Social influence | 3.36 | 1.01 |
Table 6 shows the strongest factor is perceived prestige with an average mean of 3.61 and the least strong is perceived encouragement with an average mean of 2.97. The average is 3.36 which is higher than the mean value of 3.
Table 7: Analysis of Security Risk
Statements | Mean | Std. Deviation |
I would not feel secure conducting stock trades via online stock trading systems. | 2.70 | 1.212 |
I am worried that others might be able to access my online stock trading account. | 2.91 | 1.106 |
I would not feel secure sending sensitive information across online stock trading systems. | 3.00 | 1.074 |
d not feel very safe providing personal information over online stock trading systems. | 3.06 | 1.155 |
Security Risk | 2.91 | 4.549 |
Table 7 shows the most perceived security risks are perceived loss of information theft and perceived fear of identity theft with an average mean of 3.06 and the least perceived security risk is insecurity to carry online trading with an average mean of 2.70. The average is 2.91, which is just higher than the mean value of 3.
Table 8: Analysis of Economic Risk
Statements | Mean | Std. Deviation |
I am uneasy about using online stock trading because I may lose money due to incorrect operation. | 2.841.193 | |
I am uneasy about using online stock trading because I may lose money due to a careless mistake. | 2.981.154 | |
I am uneasy about using online stock trading because I may lose money due to system processing errors. | 2.901.121 | |
en transaction errors occur, I am concerned that the securities broker may not compensate my loss. | 3.00 | 1.165 |
Economic Risk | 2.93 | 1.15 |
Table 8 shows the most perceived economic risk is the perceived denial of compensation by the broker with an average mean of 3 and the least perceived economic risk perceived fear of losing money due to incorrect operations with an average mean of 2.84. The average is 2.93, which is higher than the mean value of 3. This shows that most people are not afraid of the economic issues associated with online stock trading.
Table 9: Analysis of Functional Risk
Statements | Mean | Std. Deviation |
I may not be able to perform well on online stock trading systems because of the limited processing power of online devices. | 2.92 | 1.008 |
I am uneasy about using online stock trading because transactions may fail due to the unstable nature of online devices/operating systems/networks. | 2.94 | 1.103 |
I may not be able to perform well on online stock trading systems because of system failure. | 3.02 | 1.099 |
I am concerned that online stock trading services cannot meet my needs due to poor functionality or system malfunctions. | 2.82 | 1.109 |
Functional Risk | 2.92 | 1.07 |
Table 9 shows the most perceived functional risk is the unstable nature of operations networks with an average mean of 3.02 and the least perceived functional risk is system malfunction with an average mean of 2.82. The average is 2.92, which is just higher than the mean value of 3.
Table 10: Analysis of Behavioral Intention
Statements | Mean | Std. Deviation |
I intend to use online stock trading in the future. | 3.32 | 1.166 |
I will use online stock trading for my stock trading needs. | 3.35 | 1.015 |
I will prefer online stock trading over offline stock trading | 3.23 | 1.188 |
Behavioral Intention | 3.3 | 1.123 |
Table 10 depicts the investor’s intention to adopt online stock trading in Nepal. The strongest opinion came that investors will use online stock trading for future needs with an average mean of 3.35 and the least strong opinion came for investor’s future intention to use online stock trading with a mean value of 3.32. The average is 3.3, which is higher than the mean value of 3.
Table 11: Correlation Analysis
PE | EE | SI | SR | ER | FR | BI | ||||||
PE | 1 | .778** | .687** | .338** | .416** | .378** | .721** | |||||
EE | 1 | .743** | .481** | .399** | .505** | .707** | ||||||
SI | 1 | .537** | .423** | .439** | .598** | |||||||
SR | 1 | .578** | .534** | .552** | ||||||||
ER | 1 | .649** | .511** | |||||||||
FR | 1 | .506** | ||||||||||
BI | 1 | |||||||||||
** Correlation is significant at the 0.01 level (2-tailed).
Note: Number of Observation N is 89, PE- Performance Expectancy, EE Effort Expectancy, SI- Social Influence, SR Security Risk, ER- Economic Risk, FR Functional Risk, and BI Behavioral Intention Performance Expectancy and Effort Expectancy have a high degree of positive correlation with Behavioral Intention, while Social Influence, Security Risk, Economic Risk, and Functional Risk have a moderate degree of positive correlation with Behavioral Intention.
|
||||||||||||
Table 12: Regression Analysis
Model | Constant | PE | EE | SI | SR | ER | FR | R square | F | Sig |
1 | -0.260 | 0.399* | 0.329* | -0.81 | 0.232 | 0.175 | -0.33 | 0.672 | 23.912 | 0.000 |
(0.419) | (0.002) | (0.019) | (0.521) | 0.027) | (0.085) | (0.767) | ||||
|
(3.017) | (3.653) | (2.661) | (1.980) | (2.278) | (2.131) |
Note: * Results are significant at a 5 percent level of significance, ** Results are significant at a 1 percent level of significance. The figure in the parentheses indicates the level of significance and variance inflation factor (FIV) respectively.
PE- Performance Expectancy, EE Effort Expectancy, SI- Social Influence, SR Security Risk, ER- Economic Risk, FR Functional Risk, and BI Behavioral Intention
Table 17 revealed that the model is fitted at a 5% level of significance as indicated by the P-value of 0.000. The coefficient of Determination (R2) is 0.672 which shows that in this study the independent variables explained 67.2% of the variations in the dependent variable. The remaining 32.8 % represents another proxy of independent variables that were not considered in this study
Model Diagnostic Test
The variance Inflation Factor of all independent variables (VIFPE =3.017, VIFEE =3.653, VIFSI= 2.661, VIFSR = 1.980, VIFER = 2.278, VIFRR = 2.131) is less than 5. So, the model is free from Multicollinearity.
CONCLUSION
This paper measures the relationship between performance expectancy, effort expectancy, social influence, security risk, economic risk, functional risk, and investor’s intention to adopt online stock trading. It is found that performance expectancy and effort expectancy are positively and significantly related to behavior intention. Likewise, social influence and functional risk have a negative insignificant relationship with behavior intention similarly security risk and economic risk have a positive insignificant relationship with behavior intention. However, they seem to ignore the risk factors associated with it as the security risk and economic risk did not show a significant impact on investor’s intention to adopt online stock trading. Maybe investors are only seeing the money that can be earned from stock trading but ignoring how risky it can be, given there is the chance of losing personal information and also the money because of the small and silly mistakes. Hence, if the online stock trading is made safe, secure, and convenient, both the parties associated i.e. regulator bodies and investors can benefit which ultimately will benefit the economy as a whole.
REFERENCES
Bagozzi, R. P., & Dholakia, U. M. (2002). Intentional social action in virtual communities. Journal of Interactive Marketing, 16(2), 2-21.
Constantiou, I. D., & Mahnke, V. (2010). Consumer behavior and mobile TV services: Do men differ from women in their adaptation intentions? Journal of Electronic Commerce Research, 11(2), 127-139.
Damghanian, H., Zarei, A., & Kojuri, M. A. (2016). Impact of perceived security on trust, perceived risk, and acceptance of online banking in Iran. Journal of Internet Commerce, 15 (3), 214-238.
Hou, J. (2015). Online stock trading: Do demographics, Internet usage, and attitudes matter? International Journal of Business and Social Science, 8
Maharjan, M. (2018). Citizens’ acceptance of e-Government services in Nepal: applying UTAUT model. International Journal of Advanced Social Sciences, 1(2), 23-32.
Poudel, B. R. (2018). Online recruitment: A cognitive perspective of job seekers in Nepal. Journal of Business and Social Sciences, 1-17.
Talukder, M. (2012). Factors affecting the adoption of technological innovation by individual employees: An Australian study. Procedia – Social and Behavioral Sciences, 40, 52-57.
Thaker, M. A., Thaker, H. B., Rahman, M. P., Amin, M. F., Pitchay, A. B., & Olaniyi, N. O. (2019). Factors affecting investor’s intention to invest in a peer-to-peer lending platform in Malaysia: An extended technology acceptance model. ADBI Working Paper Series, 1-17.
(Srijana Gyawali, an MBA Scholar at Tribhuvan University, Lumbini Banijya Campus, can be reached at [email protected])
Related News
Trending
-
1
Legendary wisdom of “Akbar & Birbal” (Photo Feature)
-
2
Students celebrate 22nd National Paddy Day (Photo Feature)
-
3
From Forests to Feeds: How AI made Bigfoot & Yeti social media stars? (Video)
-
4
Aarati at ISKCON Temple (Photo Feature)
-
5
Sarina Weigman-Torchbearer of Dutch Women’s Football Team
-
6
Bungamati and Khokana keep paddy plantation tradition alive amid Urban Sprawl (Photos)
-
7
Farmers celebrate 22nd National Paddy Day (Photos)
News Update
-
Flood cuts access to Kuseshwor Mahadev Temple in Sindhuli (Photo Feature)
-
Nepal wins ACC U-16 East Zone Cup title for consecutive second time
-
Bridge on Banepa–Nala Road Collapses (Photos)
-
Abandoned Villages on Kathmandu’s Outskirts: A Tale of Urban Migration (Photos)
-
B.P. Highway in critical condition (Photo Feature)
-
Maharajgunj-Chappal Karkhana Road expansion at a snail’s pace (Photos)
-
NDDRMA coordinates drone training for 3 teams of security agencies (Photos)